The study of complex networks has attracted more and more interest in the recent past, fueled in particular by the development of communication and information networks. It turned out that many important aspects of the physical world or of social interactions can also be modelized by such networks. However, these powerful tools have never been applied to the study of games.
Games have been played for millenia, and besides their intrinsic interest, they represent a privileged approach to the working of human decision-making. They can be very difficult to modelize or simulate: only recently were computers able to beat chess champions. The old Asian game of go is even less tractable, as no computer program has been able to beat a very good player.
We have performed the first study of the game of go from a complex network perspective. We built a directed network which reflects the statistics of tactical moves. Study of this network for data sets of professional and amateur games shows that the move distribution follows Zipf’s law and the network is scale free, with statistical peculiarities different from e. g. the World Wide Web. These specificities reflect in the outcome of ranking algorithms, such as the PageRank at the basis of the Google search engine. The fine study of eigenvalues and eigenvectors of the matrices used by the ranking algorithms singles out certain strategic situations (see figure), and vary between amateur and different professional tournaments. These results should pave the way to a better modelization of board games and other types of human strategic scheming.
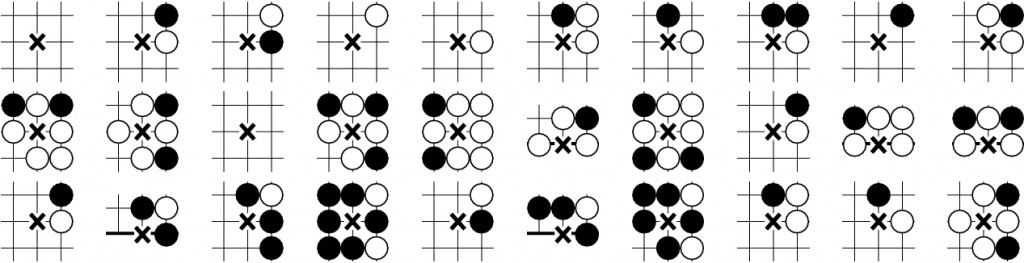
See B. Georgeot and O. Giraud , EPL 97 68002 (2012), and CNRS highlight (in French) here